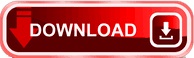
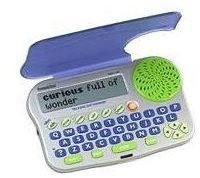
Most commonly, the edit operations allowed for this purpose are: (i) insert a character into a string (ii) delete a character from a string and (iii) replace a character of a string by another character for these operations, edit distance is sometimes known as Levenshtein distance. Take an example as, Given two character strings s1 and s2, the edit distance between them is the minimum number of edit operations required to transform s1 into s2. In Naive Bayes algorithm, we calculate the edit distance between the query term and every dictionary term, before selecting the string(s) of minimum edit distance as spelling suggestion. There is some more algorithm which is based on Edit distance like Naive approach, Peter Norvig, Symmetric Delete Spelling Correction.

If possible, construct an error model of common mistakes.Ī spelling correction tool can help improve users’ efficiency in the first case, but it is more useful in the latter since the users cannot figure out the correct spelling by themselves. For your example, you need to construct a custom dictionary of all possible words and a corpus reflecting the frequency of occurrences for the custom dictionary words. A channel model reflects if an error happens depending on how the word is transmitted. In case of rank and their score candidate replacement type of spelling error detection, we use some probabilistic model like language model in which will find the weights how likely a word will appear in the current context. In case of generation of candidate suggestions, we need to find dictionary words that are similar to an incorrect word and typically similarity measure on the basis of edit distance (e.g replaces, insert of individual characters, transpose etc. Otherwise, we need to build a separate model which help to find the given word is potentially incorrect on the basis of their current context. In detecting the incorrect word the simplifying assumption is that if any word which is not in a dictionary is a spelling error. In spelling correction there are many ways to detect like detect an incorrect word, generate candidate suggestions, giving the rank according to their score in the candidate replacement. We mention them here for the sake of completeness. Or you are trying hard to fit in everything within a text message or a tweet and must commit a spelling sin. Short forms/Slang: In this case may be u r just being kewl. Non-word Errors: This is the most common type of error like if we type langage when you meant language or hurryu when you meant hurry. Or if you type in three when you meant there. E.g, typing buckled when you meant bucked.
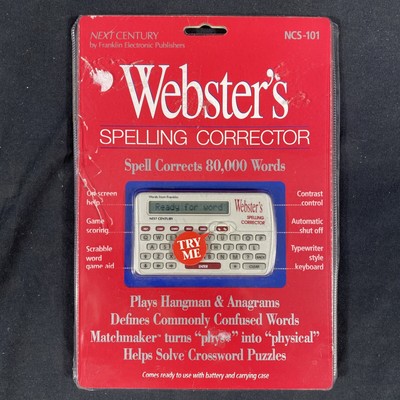
Real Word Errors: Sometimes instead of creating a non-word, you end up creating a real word, but one you didn’t intend. Cognitive Errors: In this type of error the words like piece-peace knight-night, steal-steel are homophones (sound the same). There are different types of spelling errors. There is not much difference between the two in theory. So, in practice, an autocorrect is a bit more aggressive than a spellchecker, but this is more of an implementation detail - tools allow you to configure the behavior. In case of the correct word already having been typed, the same is retained. An auto corrector usually goes a step further and automatically picks the most likely word. Spelling Errors, most of the time for spelling correctness checked in the context of surrounding words. Firstly, I will explain some other model architecture which is also used in Natural Language Processing task like speech recognition, spelling correction, language translation etc. Basically, spelling correction in natural language processing and information retrieval literature mostly relies on pre-defined lexicons to detect spelling errors. In this article, I will use bi-direction LSTM in the encoding layer and multi-head attention in the decoding layer. Tensorflow, Sequence to Sequence Model, Bi-directional LSTM, Multi-Head Attention Decoder, Bahdanau Attention, Bi-directional RNN, Encoder, Decoder, BiDirectional Attention Flow Model, Character based convolutional gated recurrent encoder with word based gated recurrent decoder with attention, Conditional Sequence Generative Adversarial Nets, LSTM Neural Networks for Language Modeling
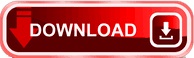